LLM to RAG: Decoding AI jargon matters, here’s why
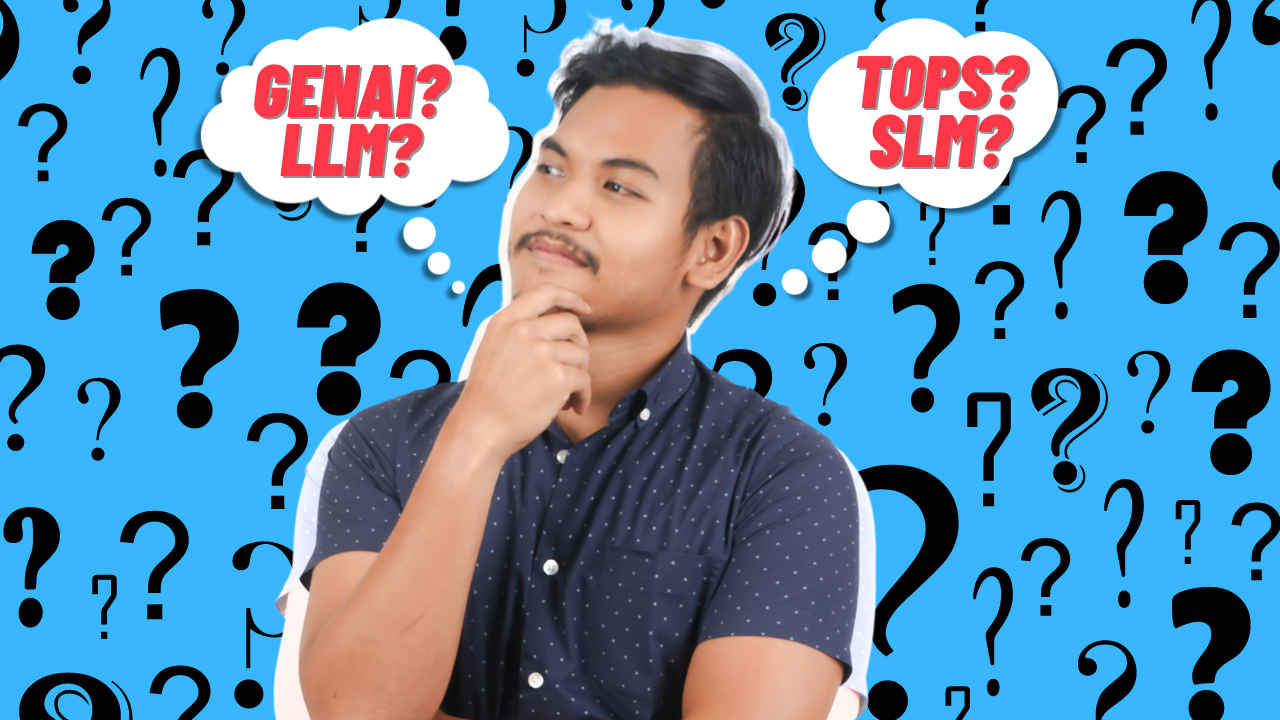
Whenever a new facet of technology debuts and gathers momentum, it introduces lots of new terminology into the lexicon. From tuning into FM radio to streaming music on-the-go, STD phone calls in the past to emoji and GIF-enabled chats in your favourite app, for example, haven’t we all learned, unlearned, and relearned quite a few tech terms over the years? Now it’s AI’s turn to introduce a whole new wave of words for us to throw around.
Naturally, as the use of AI proliferates, so does its related jargon. It’s important to address some of that jargon to avoid unnecessary confusion and to save our own sanity. I think there have been too many acronyms floating around and key concepts unexplained as far as AI is concerned. I’ve been guilty of not paying enough attention to it, something that I’m trying to correct through this article. Better late than never, right?
Also read: How RAG boosts LLM accuracy and reduces AI hallucination
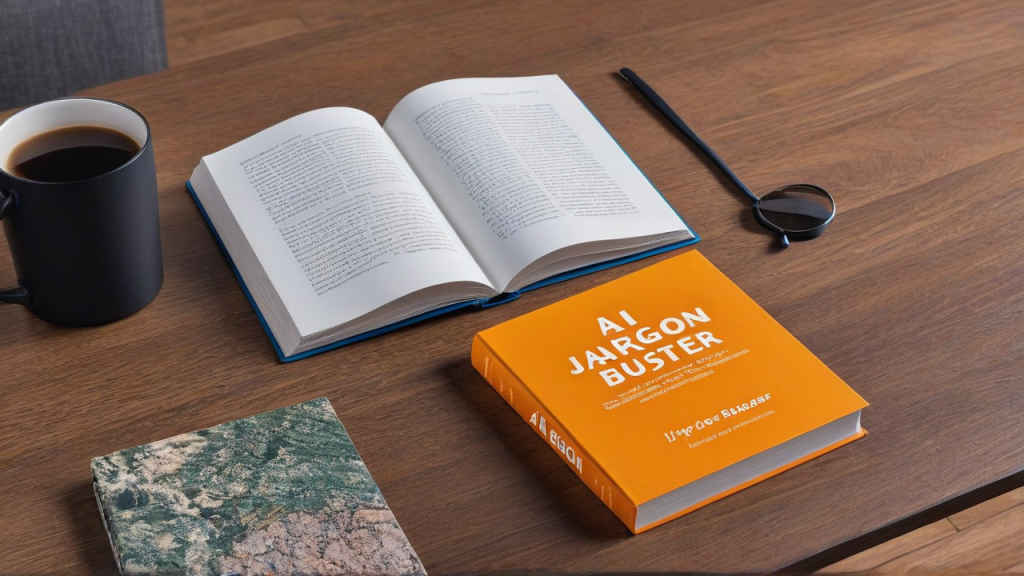
Take LLM or Large Language Models, for instance – the reason behind the current AI renaissance we are all experiencing. It’s the backbone of AI chatbots like OpenAI’s ChatGPT, Microsoft Copilot, or Google Gemini, but how many of us had even heard of such a term called LLM even five years ago? Simply put, it’s an AI algorithm trained on vast amounts of text and natural language-related data to generate textual output similar to a human would. But what about text-to-image generation services like Midjourney, Stable Diffusion, or DALL-E? They use LLMs for language demystification, but they’re strictly AI diffusion models – machine learning algorithms that progressively add noise to data and learn to reverse the process, especially useful for generating images, videos, and sound. LLMs and diffusion models make up most of the Generative AI products and services you’ve seen and used since 2022. It’s not even been two years since ChatGPT 3 blew the AI floodgates open for the general public, and we’ve already abbreviated Generative AI to GenAI. Keeping it snappy!
Then there are slightly more fundamental concepts of AI that are good to know, especially in the wake of AI PCs. Whether it’s today or in the near future, conversations on AI PC or AI Laptop won’t be complete without the mention of TOPS – something you must’ve seen across all the recent AI hardware-related articles here in the magazine or on Digit.in. TOPS, or Tera Operations Per Second, is a measure of how many trillion operations a processor can perform every second. In the context of AI hardware, TOPS is a benchmark of processing power, particularly relevant for tasks involving deep learning and neural networks. Higher TOPS indicate a more capable processor, one that can handle complex AI computations with ease. As we start reviewing and writing about AI Laptops, you’ll see TOPS being mentioned as a key parameter going forward.
Tokens, on the other hand, are a fundamental concept in the software side of AI, particularly when dealing with LLMs or SLMs (Small Language Models). The human brain learns and understands language differently from a computer, which only understands 0s and 1s. Tokens are the numerical pieces of text – words, characters, or subwords – that an AI model processes to understand and generate language. They are the individual units that make up the sentences and paragraphs produced by models like ChatGPT. The more tokens a model can process efficiently, the better it performs in generating contextually accurate text for any given scenario, based on its prompt relevance.
A prompt, of course, is another new AI-related term – quite similar to the idea of a keyword in the traditional search engine setting, but allowing for far more complexity and nuance than a keyword ever can. Hallucination is another term you will encounter a lot with respect to one of the fundamental faults in AI (LLM & GenAI) that researchers and AI companies are trying to fix – hallucination occurs when an AI model generates incorrect or misleading information presented as fact. One of the ways to reduce hallucination in LLMs is called RAG (Retrieval Augmented Generation), which is a technique to improve the factual accuracy of generative AI content by referencing outside information that wasn’t previously part of the original AI training database.
Terms like “streaming,” “Wi-Fi,” and “Bluetooth” were once new and confusing, but today they are part of our everyday vocabulary.
Of course, this is nowhere close to even scratching the surface of AI related jargon. Learning the AI jargon may seem daunting at first, filled with unfamiliar acronyms and complex concepts. However, remember that the same was true for all the early consumer tech jargon 20-25 years ago till now. Terms like “streaming,” “Wi-Fi,” and “Bluetooth” were once new and confusing, but today they are part of our everyday vocabulary. As technology progresses and evolves, our capacity to absorb and speak tech improves, making these initially bewildering terms second nature over time.
When it comes to AI, keeping up with the jargon is more than just a necessity – it’s an invitation to be part of the conversation on a more geeky level. As we continue to navigate the fast evolving AI landscape, familiarising ourselves with terms like TOPS, tokens, and prompts is just the beginning. Digging deeper and further understanding concepts such as model training, inference, neural networks, and the difference between generative and discriminative models will be crucial for anyone looking to stay informed and engaged, and fundamentally have a deeper appreciation of tech and its application in our lives.
Also read: AI hallucination in LLM and beyond: Will it ever be fixed?
Jayesh Shinde
Executive Editor at Digit. Technology journalist since Jan 2008, with stints at Indiatimes.com and PCWorld.in. Enthusiastic dad, reluctant traveler, weekend gamer, LOTR nerd, pseudo bon vivant. View Full Profile